Dr. Manu Mehta and Mr. Akhilesh Kumar
This recent study at Indian Institute of Remote Sensing (IIRS), ISRO, presents the first known comprehensive global validation of Aerosol Optical Thickness (AOT) measurements derived from the Sentinel 2 Sen2Cor algorithm, covering a significant period of 5 years from 2018 to 2022 utilizing an extensive dataset from over 400 AERONET stations (each year) worldwide (Kumar and Mehta, 2024).
The Copernicus Program includes the Earth observation mission Sentinel 2, which is intended to take high-resolution pictures of the land and coastal waterways. With a swath width of 290 km and a repetition cycle of five days, the Sentinel-2A and Sentinel-2B satellites provide a spatial resolution of 10 m, 20 m, and 60 m. Sentinel 2, carries a Multi-Spectral Instrument (MSI), which supplies information in 13 bands covering the visible and infrared portions of the electron spectrum. We employed level 2A retrievals from the Sen2Cor atmospheric correction method in this investigation. Other than the standard bottom of the atmosphere (BOA) reflectance, Level 2 also provides an Aerosol Optical Thickness (AOT) map for each scene at 550nm. To derive AOT, Sen2Cor relies on the dense dark vegetation (DDV) pixel method developed by Kaufman and Sendra, (1988). The DDV method estimates the AOT based on the correlation of SWIR band (B12) with Blue (B2) and Red bands (B4). The detailed methodology of the retrieval process can be found in Richter et al. (2012). It could be worth mentioning here that apart from Sen2Cor, other atmospheric correction processors like ATCOR, FORCE, MAJA, iCOR etc. are also in use for retrieval of surface reflectance from Sentinel 2 data, details of which can be found in Doxani et al., (2018, 2023).
The core objective of our study has been to carry out the first comprehensive global assessment of estimated level 2A Sen2Cor AOT retrievals available from Sentinel 2. This study is important to understand how accurately can the estimated AOT be retrieved using Sen2Cor which is further utilized in level 2A surface reflectance product retrievals as well as to what degree these AOT products can be used to monitor aerosols at a fine scale. It also provides a comprehensive insight on an AOT product that is relatively new and is not yet used widely in remote sensing or atmospheric science communities.
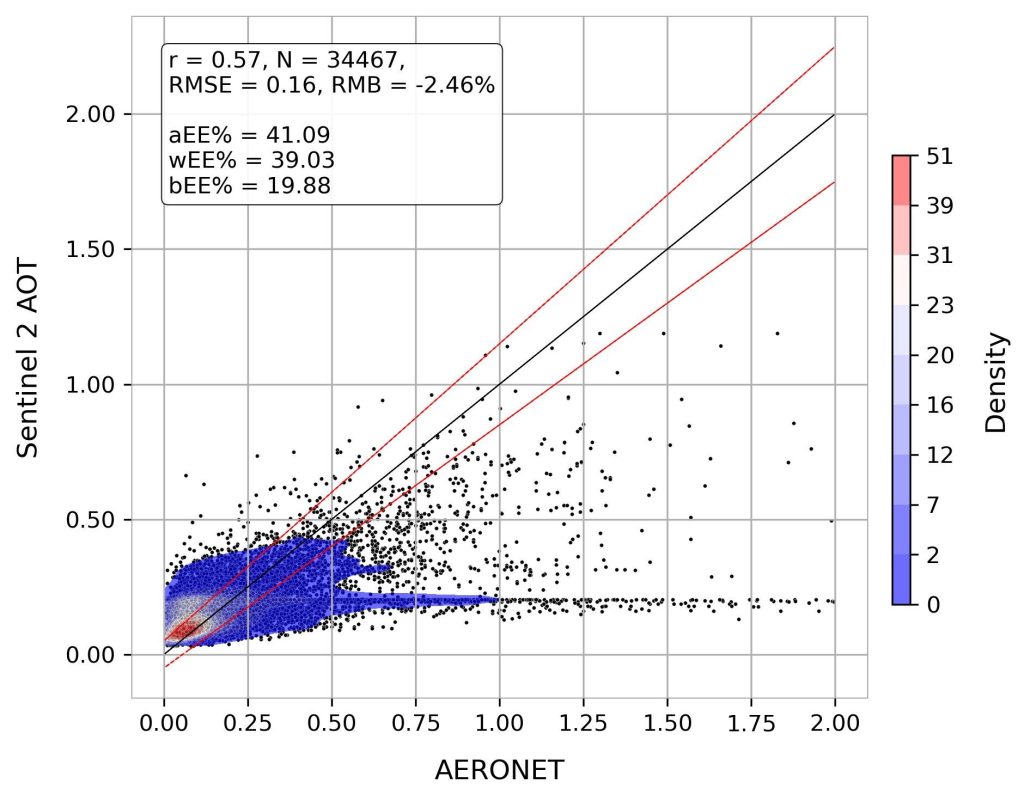
Fig. 1: Scatterplot showing AERONET and Sen2Cor derived Sentinel 2 AOT at 550nm. The top panel also shows statistics related to correlation, and RMSE, among others. Black solid line is the 1:1 line and red dotted lines are upper and lower EE bounds. (Kumar and Mehta, 2024)
We found that Sentinel 2 tends to underestimate AOT, particularly at greater aerosol loadings, according to the data. Although there appears to be a good overall correlation (r = 0.57) with low RMSE (0.16) and relative mean bias (RMB = -2.46%) between AERONET and Sentinel 2 AOT datasets (Fig.1), regional analysis reveals significant variation in performance across regions, with areas like Europe and Americas exhibiting stronger correlations and lower RMSE than others like Indian subcontinent and Southern Africa. Temporal analysis also suggests an improvement in Sentinel 2 performance, particularly in capturing extreme AOT values in 2022. Elevation does not seem to significantly affect the calculation of AOT, however minor differences may be seen across specific land cover types, such as permanent water bodies and sparse vegetation. Sentinel 2 AOT can be successfully employed for aerosol research in metropolitan areas or at a finer spatial resolution, particularly in Europe, the Mainland USA, and East Asia, however, improvements are needed across several geographical regions, such as the Indian subcontinent (Fig. 2).
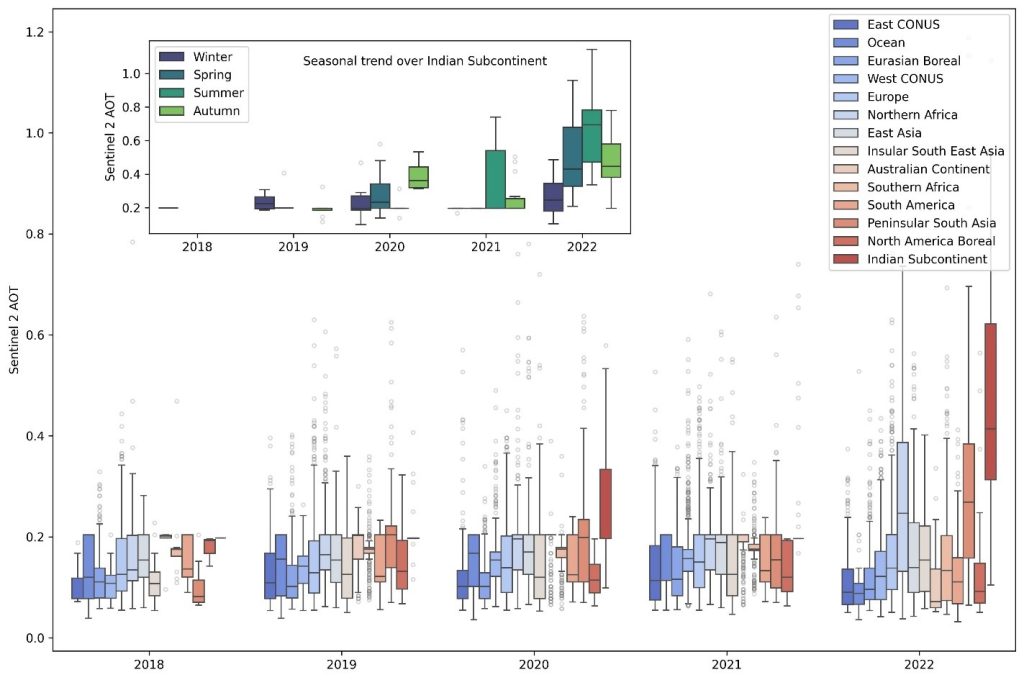
Fig. 2: Plot showing the variation in Sentinel 2 AOT across different geographical regions between 2018 and 2022. The inset plot also shows the seasonal trend over Indian subcontinent where contrary to actual conditions, Sen2Cor tends to severely underestimate AOD loadings in winter compared to summer. (Kumar and Mehta, 2024)
References
- DOXANI, G., VERMOTE, E., ROGER, J.-C., GASCON, F., ADRIAENSEN, S., FRANTZ, D., HAGOLLE, O., HOLLSTEIN, A., KIRCHES, G. & LI, F. 2018. Atmospheric correction inter-comparison exercise. Remote Sensing, 10, 352.
- DOXANI, G., VERMOTE, E., ROGER, J.-C., SKAKUN, S., GASCON, F., COLLISON, A., De KEUKELAERE, L., DESJARDINS, C., FRANTZ, D., HAGOLLE, O., and KIM, M., 2023. Atmospheric Correction Inter-comparison eXercise, ACIX-II Land: An assessment of atmospheric correction processors for Landsat 8 and Sentinel-2 over land. Remote Sensing of Environment, 285, p.113412.
- KAUFMAN, Y. J. & SENDRA, C. 1988. Algorithm for automatic atmospheric corrections to visible and near-IR satellite imagery. International Journal of Remote Sensing, 9, 1357-1381.
- KUMAR, A., & MEHTA, M. 2024. Global evaluation of sentinel 2 level 2A Sen2Cor aerosol optical thickness retrievals. International Journal of Remote Sensing, 1–17
- RICHTER, R., LOUIS, J. & MÜLLER-WILM, U. 2012. Sentinel-2 MSI – Level 2A Products Algorithm Theoretical Basis Document. Darmstadt, Germany: Telespazio VEGA Deutschland GmbH.